In the digital age, the vast amount of data generated by social media chatter, news commentary, and online discussions is a goldmine for organizations seeking to gauge public opinion. Understanding how people feel about brand initiatives, market changes, and new policies is crucial for informed decision-making. This blog post delves into the application of modern AI for extracting meaningful insights from the turmoil of social media discourse. We showcase this technique by examining the social media conversations about the ongoing labor market reforms and political strikes in Finland. By leveraging modern AI, we can decode the complexities of public sentiment, providing critical insights for businesses and policymakers aiming to understand and respond to the nuances of public opinion.
Today's decision-makers face the challenge of extracting actionable insights from extensive, unstructured social media data. This post is for you—decision-makers and innovators leveraging the latest AI advancements for strategic advantage.
We'll walk you through using large language models (LLMs), similar to those behind OpenAI’s ChatGPT, to make sense of the overwhelming social media noise. We will showcase how these techniques help you analyze and extract meaningful insights into public opinion by using social media discussions around the ongoing labor market reforms in Finland as an example. By the end of this post, you'll see how LLMs can be a game-changer in analyzing textual data, turning the massive flow of online conversations into valuable insights that can inform strategies and decisions.
The Journey from Social Media Chatter to Structured Data
The first step in our journey is data collection. This involves collecting posts, comments, and other forms of communication from various social media platforms such as LinkedIn, X (ex-twitter), and Threads. The data might also include articles and blog posts relevant to the topic of interest—in this case, Finland's labor market.Most social media platforms offer (paid) APIs for accessing the content on the platform. Alternatively, we can also find 3rd party data brokers who have already collected vast amounts of data from these platforms and others. For our analysis, we'll use a fresh dataset of tweets—approximately 3000—gathered using targeted keyword searches. Here, the keywords are crucial as they define the scope of our dataset, so we include terms directly related to the labor market discussion in Finland (see the hidden section below for a detailed list of keywords).
Once we have the raw data, the next step is to enrich it using text analytics. This is where AIs come in – to process and add layers of information to the dataset. We’ll be using two categories of techniques, which are further explained in the following chapters, to enrich the data:
- Hybrid Techniques: We employ semantic embeddings—mathematical representations of the meaning of the tweets—and combine them with statistical methods to better understand the composition of the social media chatter. This method uncovers underlying themes that might not be immediately evident, offering a richer overview of the data.
- Prompt Engineering Techniques: We explicitly instruct the AI to analyze the posts in our dataset. This includes emotion and sentiment analysis, thematic labeling, and extracting named entities like people and organizations. These techniques yield direct and interpretable results that allow us to zoom into the dataset and look at a specific topic of interest.
Once we have our enriched dataset in hand, we can start extracting insights. The enrichments—the additional layers such as emotion, sentiment, labels, and named entities—in the dataset serve as a gateway to looking at the data from various perspectives. We can isolate segments of the dataset to analyze sentiments on specific issues and reveal the nuances of the discourse. This capability allows us to extract findings from the social media chatter on different topics, providing a granular view of the public sentiment and the emotional undercurrents driving the discourse.
In the following sections, we will explore the enrichment techniques in more detail.
Hybrid Technique: Semantic Classification
Semantic classification is a sophisticated technique that marries AI's cutting-edge capabilities with proven statistical analysis methods to segment textual datasets into distinct themes. This technique offers a structured approach to see the key themes in the data.
In semantic classification, we feed each message in our dataset to an embedding model–a machine learning (ML) model much akin to large language models. This ML model converts the texts into mathematical vector representations, known as embeddings, that encapsulate each text’s semantic essence – its meaning. You can find embedding models from most major players in the field, e.g., OpenAI, Google, and AWS.
These embedding vectors serve as the foundation for the subsequent statistical analysis. By grouping vectors based on their similarity, we can form distinct segments that reflect the major themes within the dataset. This is illustrated in Figure 1, where each point is a mathematical representation of one tweet in our dataset, and similarity grouping was used to form the different colored segments.
The organized overview from semantic classification is crucial for a clear and concise interpretation of the data. After forming the segments, we employ a modern AI to review each one and generate detailed narrative summaries that highlight their key contents and underlying themes.
In our analysis, the semantic classification identifies three prominent themes:
- Labor Relations and Trade Union Activities,
- Government Critique and Political Discourse, and
- Strikes and Labor Action.
These themes are visually represented by the gold, petrol, and blue points, respectively, in Figure 1.
Semantic classification is particularly beneficial as it provides a structured way to first understand the main topics at a glance and then zoom in on each category. In the following, we’ll introduce prompt engineering -based techniques to further enrich the dataset with finer details.
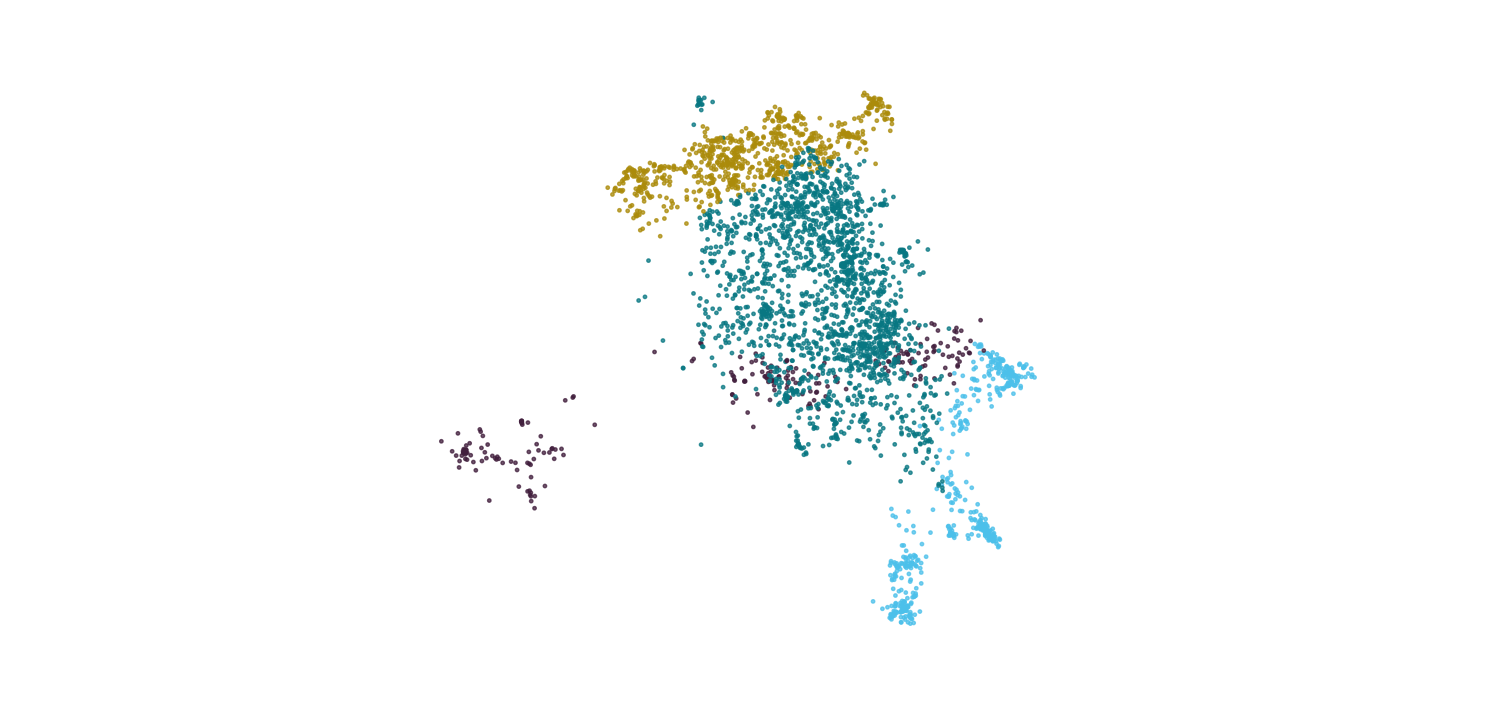
Prompt Engineering-Based Text Analysis Techniques
In text analytics, prompt engineering is a powerful strategy to harness the capabilities of modern AI for specific analyses. This approach involves writing precise instructions, prompts, that guide the AI to process and analyze text data in a desired manner.
Consider sentiment analysis as an example. We want the AI to determine whether the tone of the text is positive, negative, or neutral. To illustrate, here's a simple prompt designed to direct an LLM to perform sentiment analysis:

The same kind of prompt engineering can be extended to other forms of analysis, such as topic labeling, emotion analysis, and named entity recognition. Each analysis type enriches the dataset, allowing us to analyze it from various perspectives.
To facilitate these types of analyses systematically, we typically set up a software suite or an analysis framework for our clients, enabling the automatic application of various prompt-engineering analyses to their datasets. This offers a high ROI, since prompt-engineering-based analyses are typically quick to implement, and the approach is easily applied to new and emerging viewpoints. By simplifying the complexities of text analysis, prompt engineering enables us to extract meaningful insights with minimal manual intervention.
Insights and Implications: Public Discourse on Current Labor Market and Political situation
Leveraging the techniques outlined previously, analysis of the social media data yields a comprehensive synthesis of the discourse on the ongoing labor market and political situation in Finland. Much of this synthesis is achieved without manual human intervention, showcasing the potential of modern AI in text analysis applications.
Applying semantic classification analysis, we find the social media discussions to revolve around three major themes:
- Labor Relations and Trade Union Activities
Discussions concerning labor relations in Finland, including strikes, trade union activities, and the economic impact of labor disputes. Debates on the rights of workers, the influence of employer organizations, and the government's role in shaping labor policies. - Government Critique and Political Discourse
Political discourse with a critical view of the Finnish government's actions and policies. Mentions of specific politicians and critiques of their handling of economic policies, labor laws, social welfare, and the influence of business organizations on government decisions. - Strikes and Labor Actions
Discussion centered around specific strikes and labor actions, and on the political nature of these actions. Debates on the legitimacy and appropriateness of strikes, the public's perception of unions' actions, and the political affiliations of trade unions like PAM and JHL.
These themes underscore a heightened public interest and concern regarding the state of labor relations and political governance in Finland. Placed in a wider context, the discourse reflects a society that is actively engaging with the complexities of economic and labor reforms and contemplating the role of various stakeholders in shaping the future of the Finnish workforce.
Digging deeper, sentiment analysis indicates a consistent negative undertone across all key themes, as depicted in Figure 2. In the figure, the green segments represent positive sentiments, the yellow segments neutral, and the red segments represent negative sentiments. The prevalence of red illustrates the overall critical tone of the discourse.
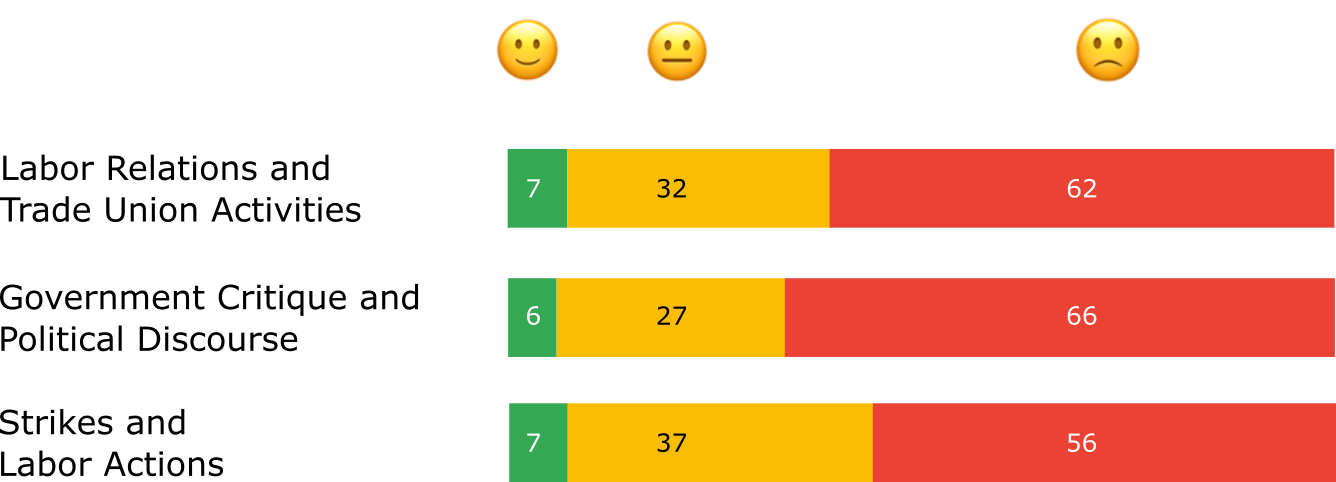
From an emotional standpoint, anger, disgust, and frustration are predominant throughout the dataset. The entities most often associated with these negative emotions, according to the dataset, are depicted in the graphic below. The size of each bubble corresponds to the frequency of negative sentiment directed at that entity, with larger bubbles indicating a higher frequency. Entities such as EK, SAK, and Prime Minister Petter Orpo appear to be significant focal points of dissatisfaction.
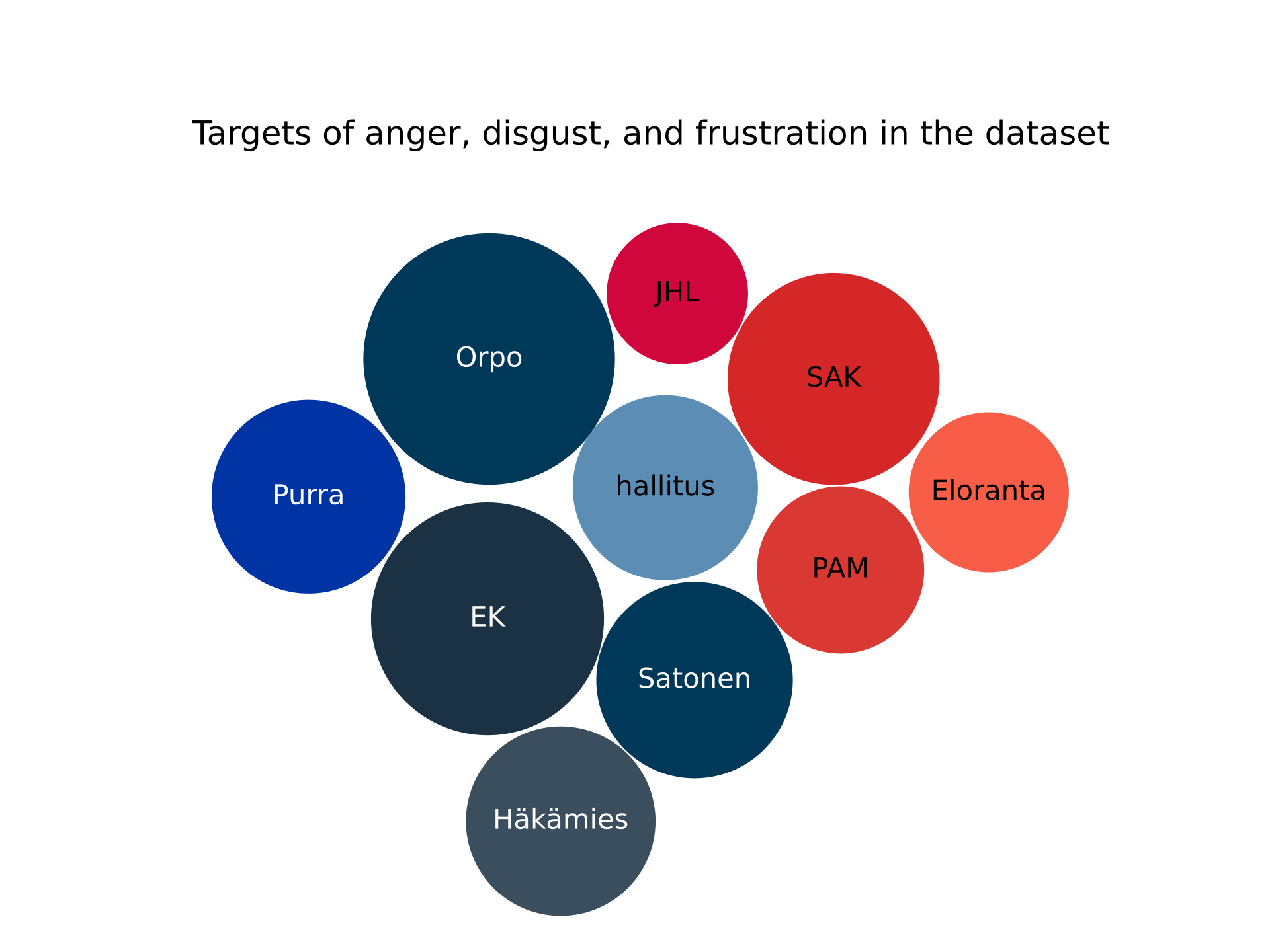
The sentiment and emotion analyses set the stage for a more detailed exploration of the underlying issues. By leveraging other enrichment dimensions in the dataset, we can delve deeper into specific topics to get a clearer understanding of the concerns and narratives that are driving these emotions. This enables us to develop interpretations of the data, potentially uncovering new and unexpected themes.
For instance, when filtering the "Labor Relations and Trade Union Activities" segment by the label ‘Economic Impact of Strikes’, we uncover a focused discourse on the financial ramifications of labor disputes:
In these messages, there is a narrative that emphasizes the significant economic costs attributed to strikes, with estimates suggesting a potential impact of nearly a billion euros. The messages reflect concerns about the negative effects on businesses and public services, which could influence the investment climate and public perception of labor unions. Discussions within this dataset also hint at a desire for labor law reforms to address these economic challenges.
AI-generated synthesis of the filtered dataset
The economic narrative varies across the three different segments. While discussions in the "Labor Relations and Trade Union Activities" segment emphasize the potential billion-euro impact of strikes, the "Government Critique and Political Discourse" segment holds a critical view of the government's fiscal policies and response to economic issues. The "Strikes and Labor Actions" segment, on the other hand, focuses on the disruptive impact of strikes on essential services and the strategic approach of unions.
Zooming in on ‘Employment law reforms’, we find a broad spectrum of opinions. On the one hand, there is a positive sentiment that underscores the importance of a dynamic economy coexisting with job security and the necessity of carefully crafted labor market reforms that potentially lead to increased employment opportunities. On the other hand, there is a strong current of negative sentiment, with users expressing opposition to government plans that may restrict political strikes and alter workers' rights, suggesting that these changes could disproportionately favor employer organizations and weaken labor protections.
The polarization of social media discussions on labor reforms and political strikes in Finland, as highlighted by the AI analysis above, mirrors a global trend. This polarization within Finnish politics has been increasing for quite some time, posing a significant barrier to productive discourse.
Embracing AI's Role in Tomorrow's Social Media Insights
We’ve highlighted the transformative impact of modern AI on text analytics, as demonstrated by our application of these advanced tools to recent social media posts about Finland's labor market discussions. These insights are not just numbers and charts—they represent the voices of thousands, distilled into a form that can guide strategies and influence policy.
For businesses, the evolving capabilities of text analytics offer:
- Competitive Advantage: Stay ahead with improved market intelligence and customer insights.
- Operational Efficiency: Streamline processes and decision-making with data-driven insights.
- Future-Ready: Prepare for sophisticated analyses as AI technologies continue to advance.
Text analytics is becoming indispensable in an information-rich world. Whether it's understanding customer sentiment, monitoring brand health, or gaining strategic market insights, text analytics can provide the edge you need. We at Brightly are ready to help you adopt these new technologies into your business. Contact us to explore the possibilities.